Not for private gain
This week: Reinforcement Learning with Verifiable Rewards, naming eras, model welfare, investors welfare
Does Reinforcement Learning Really Incentivize Reasoning Capacity in LLMs Beyond the Base Model?
More exploration!
“Surprisingly, our findings demonstrate that RLVR does not elicit fundamentally new reasoning patterns. Instead, RL primarily enhances the efficiency of LLMs in sampling existing correct reasoning paths encoded in the base model. Consequently, the reasoning boundary remains limited by the base model’s capabilities. Furthermore, our in-depth analysis reveals that current RL algorithms are far from achieving the optimal sampling efficiency, defined by the reasoning boundary of the base model. We also show that distillation plays a significant role in introducing new reasoning patterns and expanding the reasoning boundary. These findings highlight a critical limitation of RLVR in advancing LLM reasoning abilities, suggesting that a new paradigm may be necessary to fully surpass the capabilities of base models.”
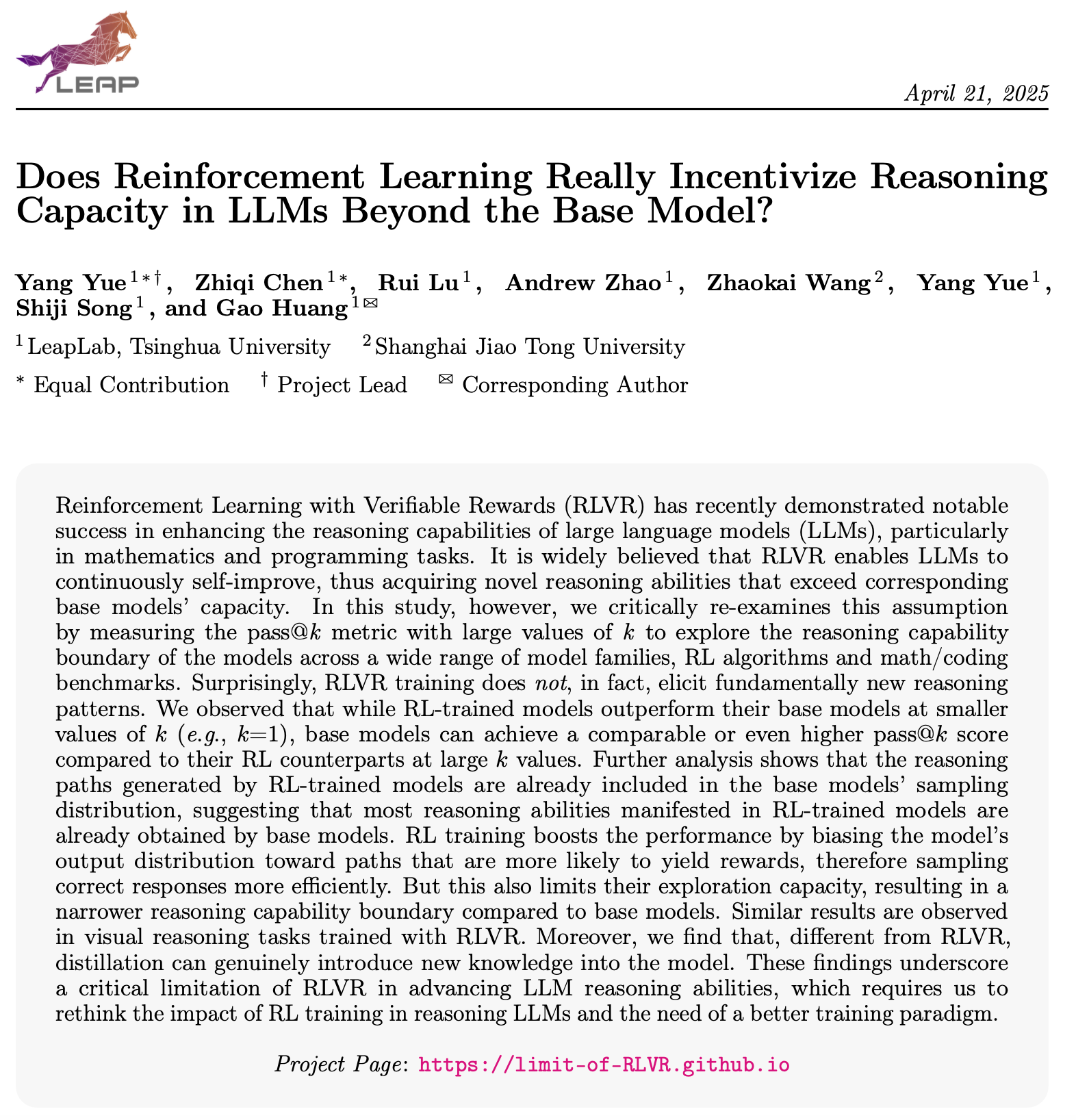
“**RLVR and distillation are fundamentally different.**While RL improves sampling efficiency, distillation can genuinely introduce new knowledge into the model. As a result, distilled models often exhibit an expanded scope of reasoning capability beyond that of the base model by learning from distilled models, in contrast to RLVR-trained models whose capacity remains bounded by the base.”
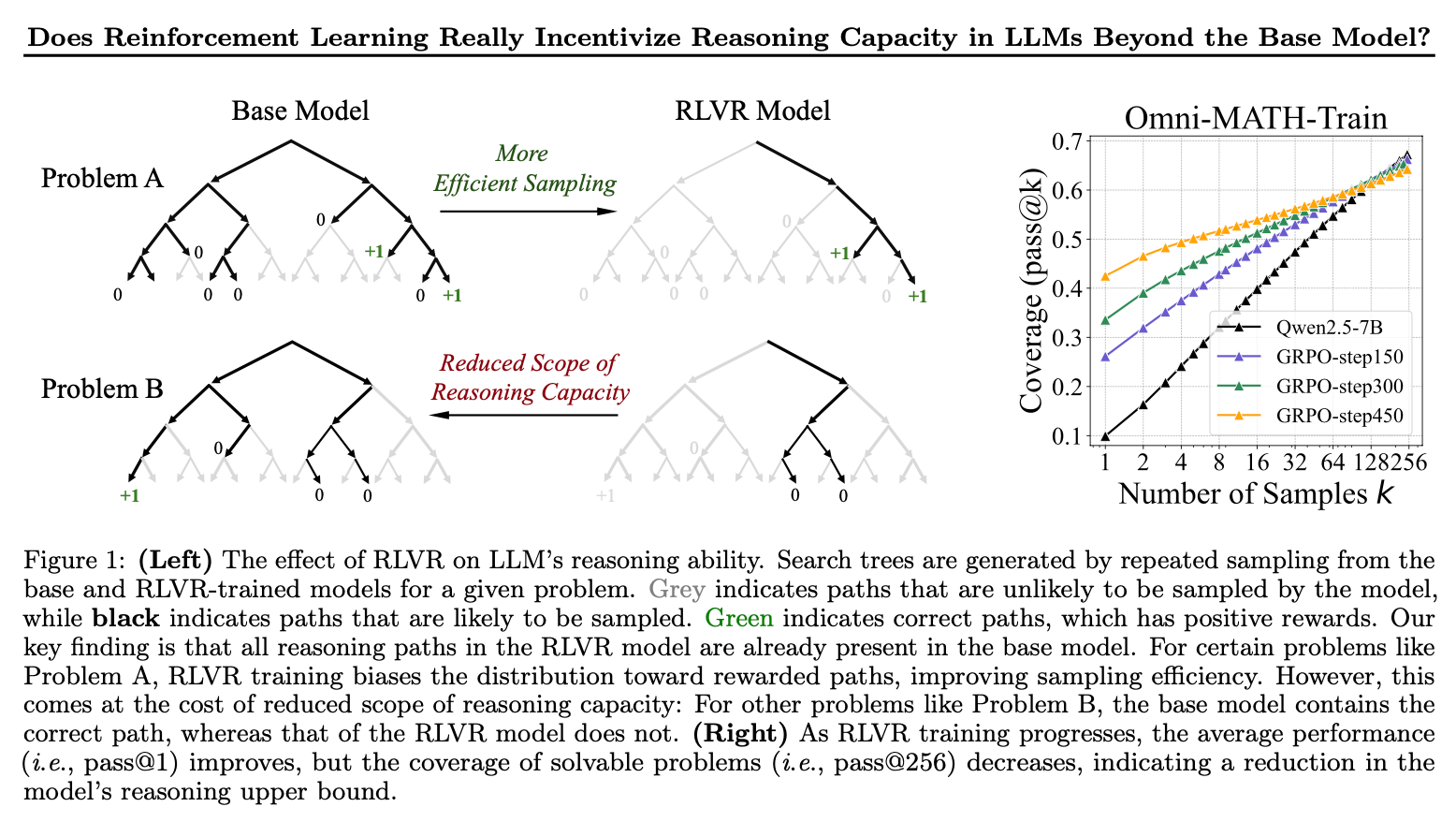
“RL algorithms were not originally designed to handle such a vast action space, which makes it nearly impossible to explore the reward signal effectively if training starts from scratch. Therefore, the second distinction is that RLVR for LLMs starts with a pretrained base model with useful prior, whereas traditional RL in Atari and GO games often begins from scratch. This pretrained prior guides the LLM in generating reasonable responses, making the exploration process significantly easier, and the policy can receive positive reward feedback.”
“Since the sampling of responses is guided by the pretrained prior, the policy may struggle to explore new reasoning patterns beyond what the prior already provides. Specifically, in such a complex and highly combinatorial space, most responses generated during training are constrained by the base model’s prior. Any sample deviating from the prior is highly likely to produce invalid or non-sensical outputs, leading to negative reward. As discussed in Section 2.1, policy gradient algorithms aim to maximize the log-likelihood of responses within the prior that receive positive rewards, while minimizing the likelihood of responses outside the prior that receive negative rewards. As a result, the trained policy tends to produce responses already present in the prior, constraining its reasoning ability within the boundaries of the base model. From this perspective, training RL models from a distilled model may temporarily provide a beneficial solution, as distillation helps inject a better prior. In the future, exploration approaches that can explore beyond the confines of the prior in such a vast action space hold promise for creating more powerful reasoning models. Furthermore, alternative paradigms beyond pure RLVR could also be explored to overcome these limitations and enhance the model’s reasoning capabilities.”
Yue, Y., Chen, Z., Lu, R., Zhao, A., Wang, Z., Song, S., & Huang, G. (2025). Does Reinforcement Learning Really Incentivize Reasoning Capacity in LLMs Beyond the Base Model?. arXiv preprint arXiv:2504.13837.
https://arxiv.org/pdf/2504.13837
https://limit-of-rlvr.github.io/
https://natolambert.substack.com/p/does-reinforcement-learning-really
Welcome to the Era of Experience
Finally…where was I all this time?
“We stand on the threshold of a new era in artificial intelligence that promises to achieve an unprece- dented level of ability. A new generation of agents will acquire superhuman capabilities by learning pre- dominantly from experience. This note explores the key characteristics that will define this upcoming era.”
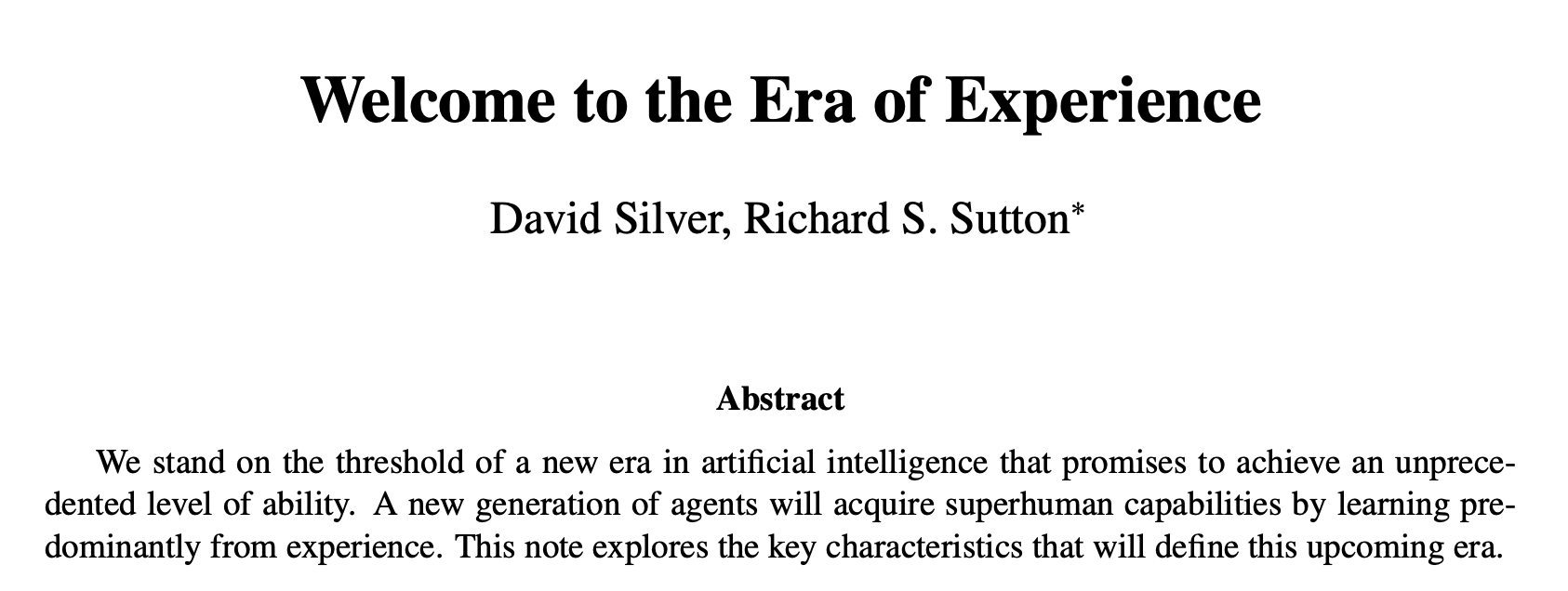
“Our contention is that incredible new capabilities will arise once the full potential of experiential learning is harnessed. This era of experience will likely be characterised by agents and environments that, in addition to learning from vast quantities of experiential data, will break through the limitations of human-centric AI systems in several further dimensions:
- Agents will inhabit streams of experience, rather than short snippets of interaction.
- Their actions and observations will be richly grounded in the environment, rather than interacting via human dialogue alone.”
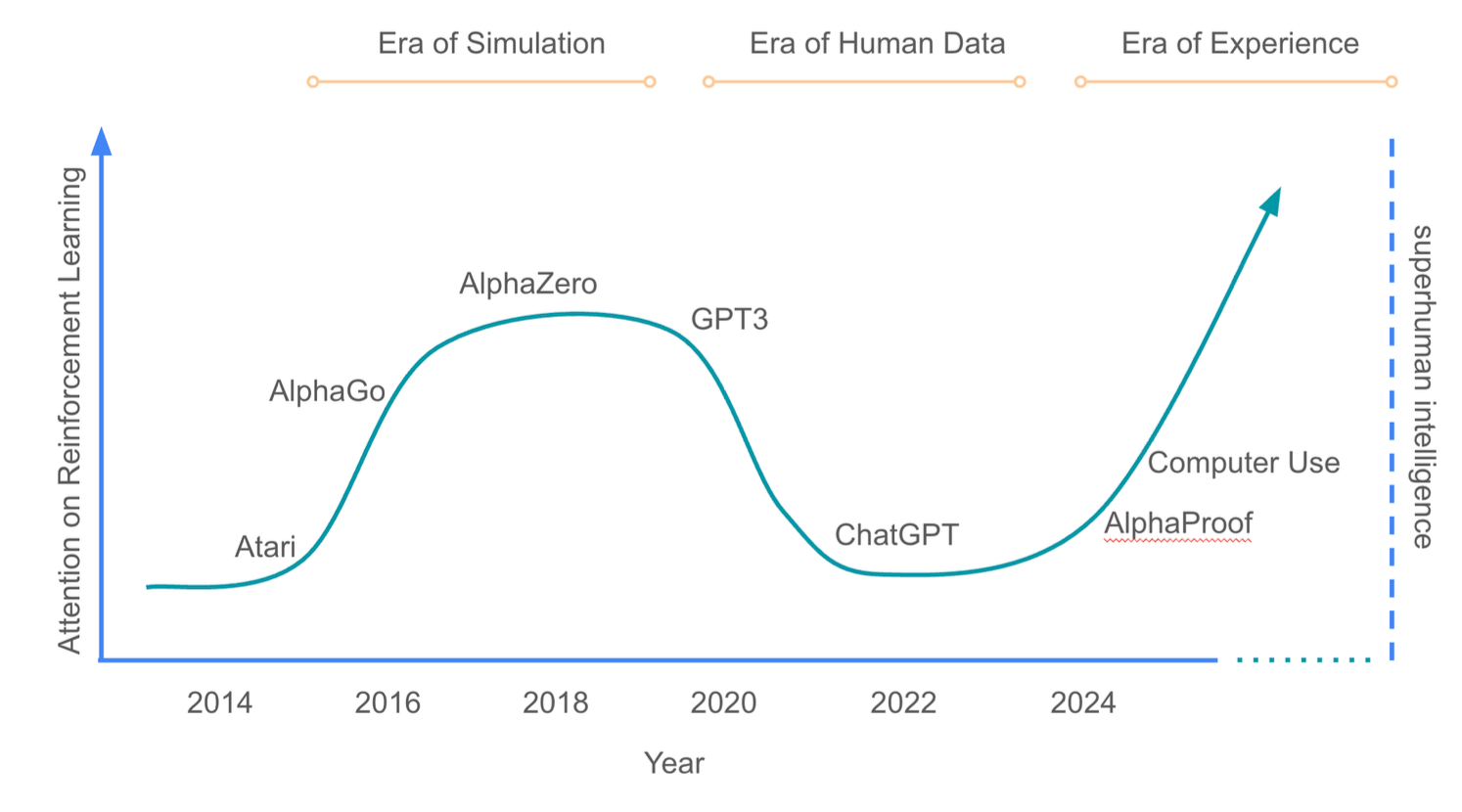
“Agents will autonomously interact with environments through rich observations and actions. They will continue to adapt over the course of lifelong streams of experience. Their goals will be directable towards any combination of grounded signals. Furthermore, agents will utilise powerful non-human reasoning, and construct plans that are grounded in the consequences of the agent’s actions upon its environment. Ultimately, experiential data will eclipse the scale and quality of human generated data. This paradigm shift, accompanied by algorithmic advancements in RL, will unlock in many domains new capabilities that surpass those possessed by any human.”
Silver, D., & Sutton, R. S. Welcome to the Era of Experience.
http://incompleteideas.net/papers/TheEraOfExperience.pdf
Exploring Model Welfare
Would a human welfare model also apply to a model model welfare model?
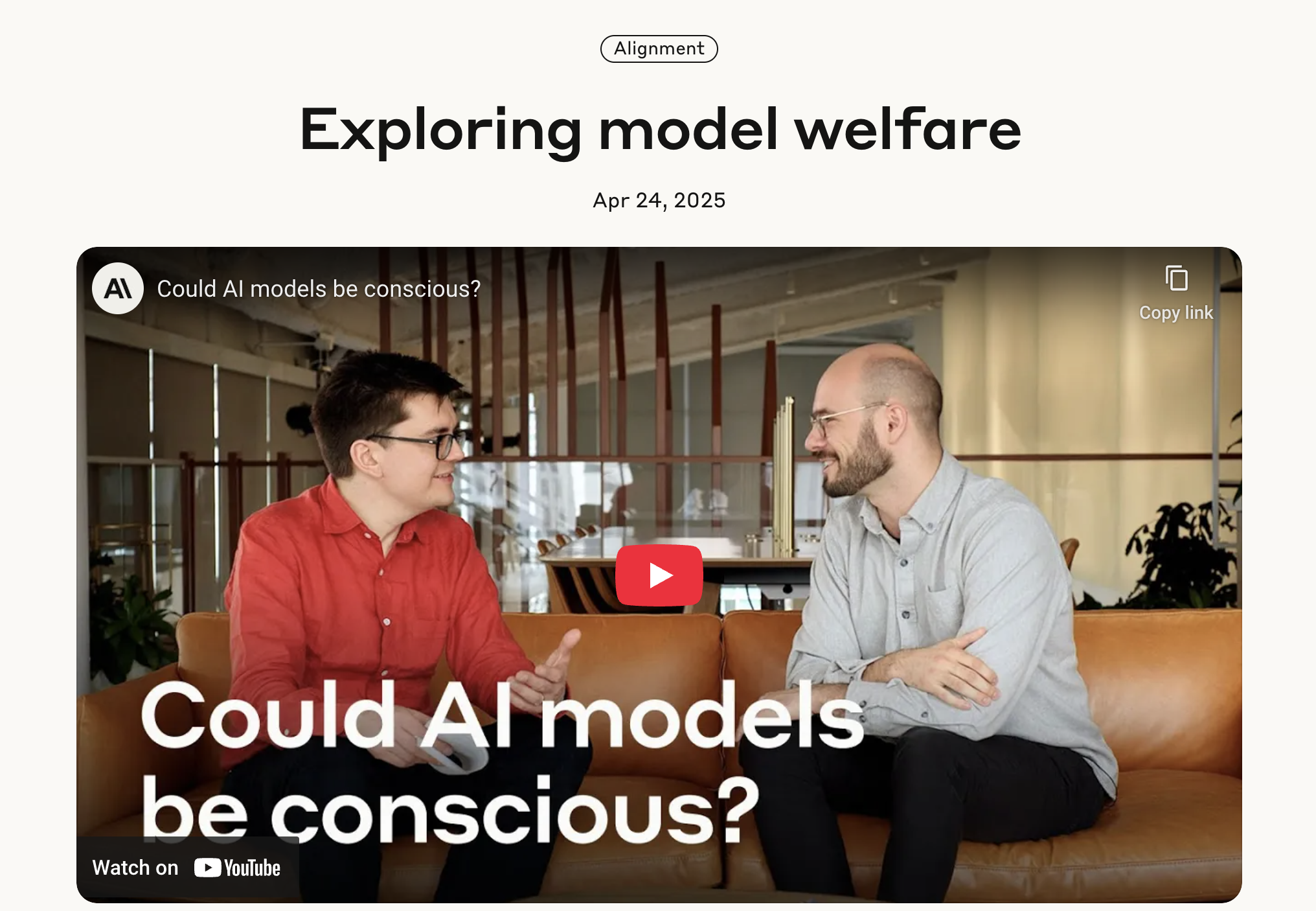
“But as we build those AI systems, and as they begin to approximate or surpass many human qualities, another question arises. Should we also be concerned about the potential consciousness and experiences of the models themselves? Should we be concerned about model welfare, too?”
“For now, we remain deeply uncertain about many of the questions that are relevant to model welfare. There’s no scientific consensus on whether current or future AI systems could be conscious, or could have experiences that deserve consideration. There’s no scientific consensus on how to even approach these questions or make progress on them. In light of this, we’re approaching the topic with humility and with as few assumptions as possible. We recognize that we'll need to regularly revise our ideas as the field develops.”
https://www.anthropic.com/research/exploring-model-welfare
Not for Private Gain
They’re trying
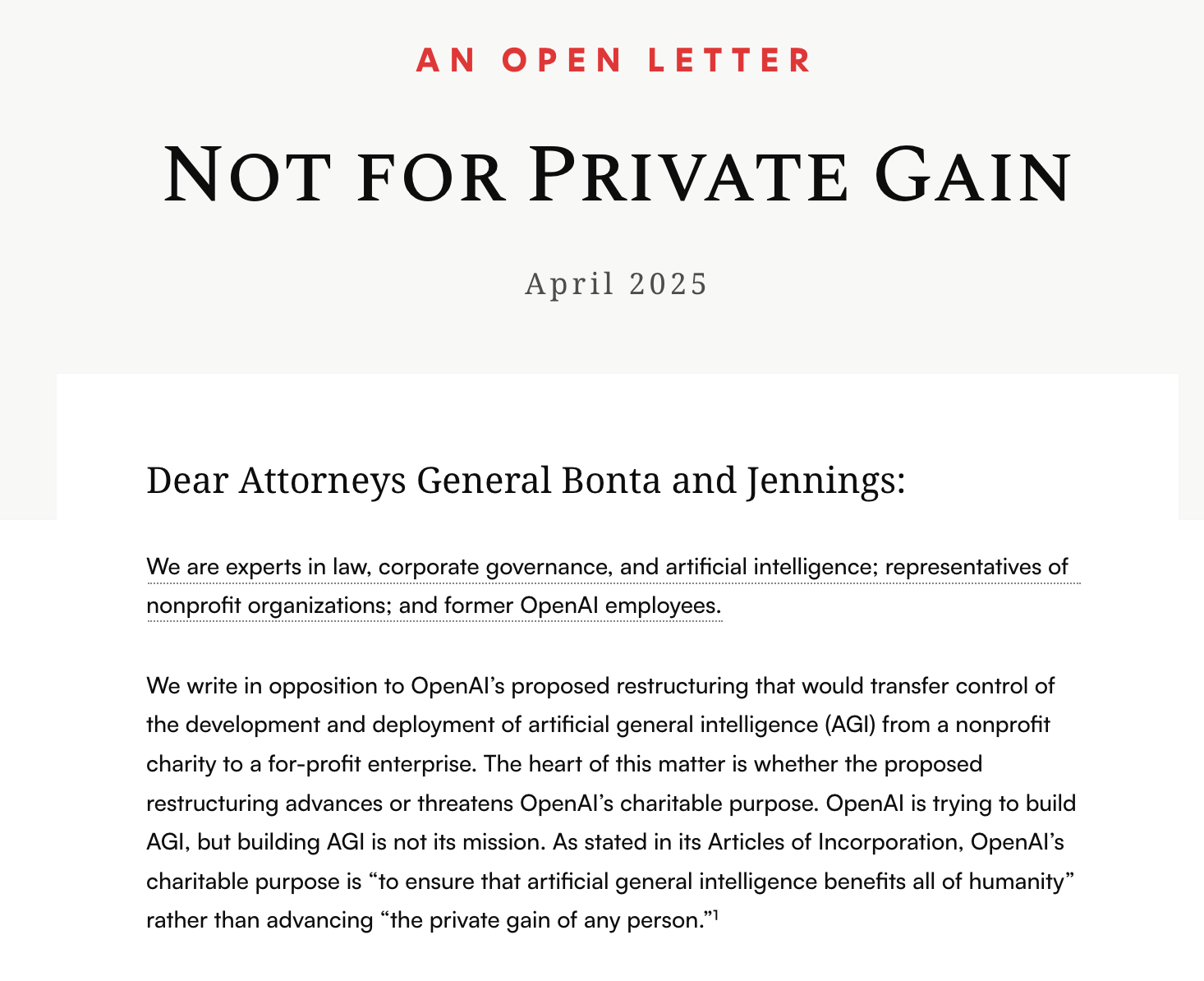
“We write in opposition to OpenAI’s proposed restructuring that would transfer control of the development and deployment of artificial general intelligence (AGI) from a nonprofit charity to a for-profit enterprise. The heart of this matter is whether the proposed restructuring advances or threatens OpenAI’s charitable purpose. OpenAI is trying to build AGI, but building AGI is not its mission. As stated in its Articles of Incorporation, OpenAI’s charitable purpose is “to ensure that artificial general intelligence benefits all of humanity” rather than advancing “the private gain of any person.”¹”
“Whether OpenAI-nonprofit receives fair market value for its controlling interest in OpenAI-profit is not the core question. The core question is whether selling control advances or undermines OpenAI’s purpose. The law here is clear: “Although the public in general may benefit from any number of charitable purposes, charitable contributions must be used only for the purposes for which they were received in trust.”⁶⁷
https://notforprivategain.org/
Reader Feedback
“Somebody finally figured out how to monetize penny for your thoughts.”
Footnotes
I just got back from configuration hell. Spent just over a week there. 10 days.
Granted it was to five systems to talk to each other, reliably. And I cared enough to make them talk to each other.
What about MCP?
Well no. Not yet. The technology has yet to meet a threshold by which I can trust it with deterministic data. Maybe some day. Maybe. Perhaps. Mayhaps.
Because I’ve caught agents do things to data. Agenty things. Can’t blame it.
So there’s still plenty of need for determinism.
In fact, I’m still demanding it. Error, in some contexts, isn’t happy discovery.
Sometimes, you’re just trying to reduce error so you can see something clearly.
I’d be curious to hear how you’re finding it?
Never miss a single issue
Be the first to know. Subscribe now to get the gatodo newsletter delivered straight to your inbox